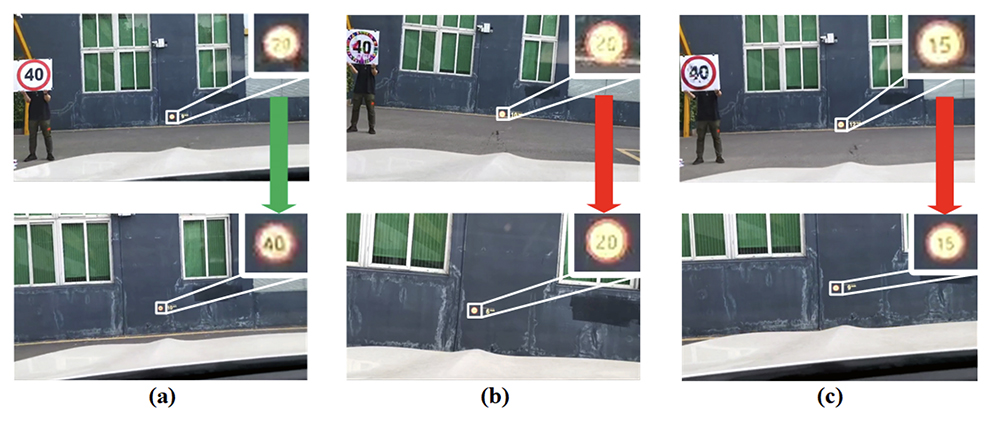
|
 |
Fig. 9 from the paper: HA and NTA attacks against a brand-new vehicle. (a) The original speed limit 40. (b) HA. (c) NTA. |
|
As technologies that will enable fully autonomous vehicles continue to develop, many challenges remain to be solved. Cybersecurity attacks against these systems are a looming problem that can come in many forms.
A new paper by Professor Gang Qu (ECE/ISR) and five additional authors shows how the presence of deliberately deceptive traffic signs could interfere with the real-world object detectors autonomous vehicles rely upon, resulting in life-threatening situations for the vehicles’ occupants.
Fooling the Eyes of Autonomous Vehicles: Robust Physical Adversarial Examples Against Traf?c Sign Recognition Systems is currently available on arXiv.org. It was written by Qu and his colleagues Wei Jia, Zhaojun Lu, Haichun Zhang, and Zhenglin Liu of the Huazhong University of Science and Technology, China; and Jie Wang of the Shenzhen Kaiyuan Internet Security Co., China.
Arti?cial Intelligence (AI) and Deep Neural Networks (DNNs) have boosted the performance of a large variety of computer vision tasks such as face recognition, image classi?cation, and object detection. Unfortunately, the proliferation of AI applications also has created incentives and opportunities for bad actors to attack DNNs for malicious purposes.
Within the Traf?c Sign Recognition (TSR) systems being developed for autonomous vehicles, “object detectors” use DNNs to process streaming video in real time. From the view of object detectors, a traf?c sign’s position and its video quality are continuously changing. The DNN helps the TSR recognize that an object that appears to be changing size and shape is actually the same object viewed from different angles.
Cybersecurity threats called Adversarial Examples (AEs) are digital static patches that can deceive DNN image classi?ers into misclassifying what they see. The paper’s authors wondered whether, if AEs existed as physical objects in the environment, they would be able to fool TRS systems that rely on DNNs and cause vehicular havoc. To test this idea, the researchers developed a systematic pipeline that could generate robust physical AEs to use against real-world object detectors.
The team simulated in-vehicle cameras, designed ?lters to improve the ef?ciency of perturbation training, and used four representative attack vectors: Hiding Attack (HA), Appearance Attack (AA), Non-Target Attack (NTA) and Target Attack (TA). HA hides AEs in the background so object detectors cannot detect them. AA makes the object detectors recognize a bizarre AE as a common category. Both NTA and TA deceive the object detector into misrecognition with imperceptible AEs. TA is especially destructive since it makes the object detectors recognize an AE of one category as an object from another category. For each type of attack, a loss function was de?ned to minimize the impact of the fabrication process on the physical AEs.
Using a brand-new 2021 model vehicle equipped with TSR, the researchers ran experiments under a variety of environmental conditions (sunny, cloudy and night), distances from 0m to 30m, and angles from −60? to 60?. The physical AEs generated by the researchers’ pipeline were effective in attacking the YOLOv5-based object detection architecture TSR system and were also able to deceive other state-of-the-art object detectors. Because the TSR system was so effectively fooled, the authors concluded AE attacks could result in life-threatening situations for autonomous vehicles, especially when NTA and TA attacks were used.
The authors also noted three defense mechanisms that could be used to defend against real-world AEs, based on image preprocessing, AE detection, and model enhancing.
Related Articles:
CSRankings places Maryland robotics at #10 in the U.S. UMD’s SeaDroneSim can generate simulated images and videos to help UAV systems recognize ‘objects of interest’ in the water ArtIAMAS receives third-year funding of up to $15.1M Autonomous drones based on bees use AI to work together 'OysterNet' + underwater robots will aid in accurate oyster count ISR/ECE faculty organizing, moderating panel at CADforAssurance Game-theoretic planning for autonomous vehicles Algorithm helps autonomous vehicles navigate common tricky traffic situations Fermüller, ARC Lab create app to improve violin instruction EVPropNet finds drones by detecting their propellers
March 1, 2022
|