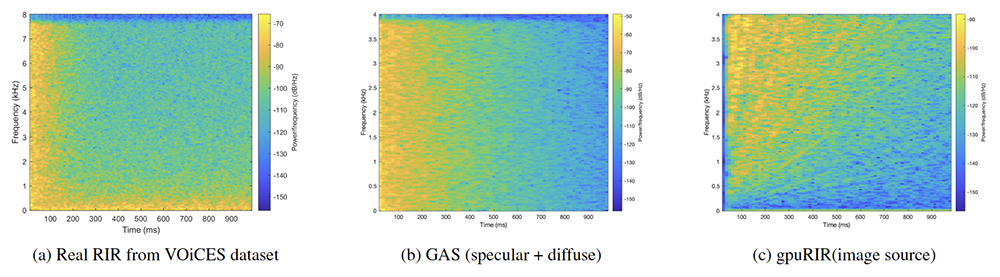
|
 |
Fig. 1 from the paper. Comparison of the spectrograms of synthetic RIRs with a real RIR from the VOiCES dataset. The approach based on GAS is more accurate when compared to the image source method used in gpuRIR.
|
|
Automatic speech recognition systems (ASR), which translate spoken words into text or instructions, have come a long way since they were first conceived in the 1950s. They are now used for applications like voice command systems, digital dictation, video gaming, voicemail-to-text and personal digital assistant devices like Alexa.
Such systems have evolved over the decades and currently are using hidden Markov models, neural networks, deep learning and other artificial intelligence techniques. One of the remaining challenges for ASR is “speaker independence,” or being able to tell the difference between multiple speakers in the same setting—often referred to as the “cocktail party problem.”
New work by ISR-affiliated Distinguished University Professor Dinesh Manocha (ECE/CS/UMIACS) and his Ph.D. students Rohith Aralikatti (CS), Anton Ratnarajah (ECE), and Zhenyu Tang (CS) introduces the idea of using reverberant acoustics within a room to help separate speakers. Their paper, Improving Reverberant Speech Separation with Synthetic Room Impulse Responses, was presented at the 2021 IEEE Automatic Speech Recognition and Understanding Workshop.
Humans are able to easily separate multiple streams of audio and speech because they use both spatial audio information as well as higher contextual cues such as the content of what is being spoken. However, ASR systems still struggle to match human performance, especially in the presence of noise and reverberation.
With recent advances in deep learning, supervised approaches to speech separation have become popular. In real-world scenarios, speech signals are reflected from the walls and objects present in the room to create reverberation effects. Most well-studied algorithms and models for source separation do not adequately account for such reverberation effects. As a result, this can impact the real-world performance of speech separation systems.
In their paper, the authors make use of curriculum learning, which corresponds to a training procedure where the model is first trained on simpler data and the model is slowly exposed to more complex data as the training progresses. The paper represents the first time curriculum learning techniques have been applied to the task of reverberant speech separation.
Pre-training is another well-known machine learning technique that has been shown to improve model robust-ness and uncertainty estimates. The authors find using a simple pre-training method causes a significant improvement in the performance of reverberant speech separation.
The team presents new techniques to train an ASR model using a mixture of synthetic and real data. They use more accurate methods to improve separation quality by focusing on the quality of synthetic realistic room impulse response (RIR) generation methods used during training. The researchers’ GAS generates impulse responses based on specular and diffuse reflections, which considerably improve accuracy. They also propose novel training procedures which seek to make better use of synthetic data to improve the real-world performance.
This combination of pre-training, curriculum learning and training on RIRs generated by an accurate geometric acoustic simulator (GAS) can significantly improve the performance of reverberant speech separation compared to an image source method baseline.
Related Articles:
‘Priming’ helps the brain understand language even with poor-quality speech signals New UMD Division of Research video highlights work of Simon, Anderson Neural and computational mechanisms underlying musical enculturation NSF funding to Fermüller, Muresanu, Shamma for musical instrument distance learning using AI New system could improve thermal devices used to check our temperatures and respiration Espy-Wilson is PI for NSF project to improve 'speech inversion' tool New methodology to estimate neural activity published by eLife Min Wu receives Senior Faculty Outstanding Research Award How does the brain turn heard sounds into comprehensible language? Wu, Ranadive developing accurate, convenient home heart monitoring system
March 9, 2022
|