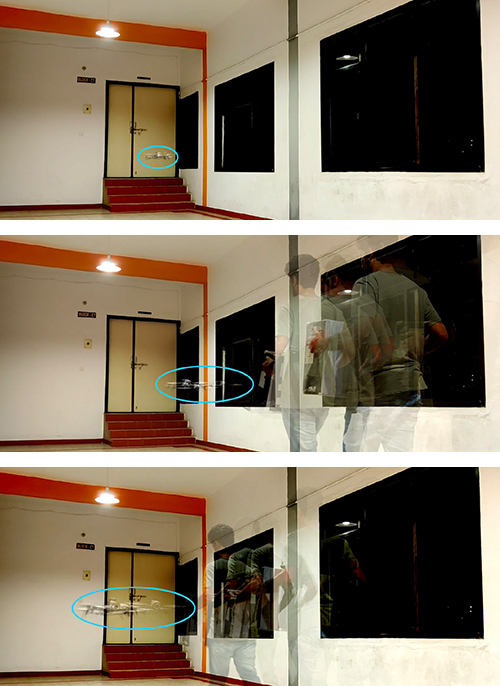
|
 |
Snapshots of the real-time collision avoidance maneuver. The snapshots show the instances of the robot sample from a non-parametric Pearson fit over experimental data. The same applies to the obstacle (human moving). Throughout the experiment, an approximate confidence (η) of 0.75 is maintained. (Fig. 6 from the paper) |
|
Reactive navigation is a well-known paradigm for controlling an autonomous mobile robot. It makes control decisions by processing current and recent sensor data, including ego-motion, the 3D motion of the robot’s camera relative to a rigid scene. To ensure robots can avoid collisions in the real world, reactive navigation requires them to explicitly consider perception and ego-motion uncertainty while computing control inputs.
Incorporating only the mean information of the uncertainty often turns to be inadequate. In contrast, bounding volume approaches are simple and induce robustness but tend to be overly conservative.
Taking uncertainty into account, roboticists would like to be able to compute low cost control inputs while ensuring some upper bound on the risk of collision. In a new paper published in the April 2020 issue of IEEE Robotics and Automation Letters, ISR-affiliated Professor Dinesh Manocha (ECE/CS/UMIACS) and his colleagues formulate these requirements in a robust Model Predictive Control (MPC) framework, wherein the robustness stems from the constraints imposed by Probabilistic Velocity Obstacle (PVO).
Reactive Navigation Under Non-Parametric Uncertainty Through Hilbert Space Embedding of Probabilistic Velocity Obstacles was written by Manocha and SriSai Naga Jyotish Poonganam, Bharath Gopalakrishnan, Venkata Seetharama Sai Bhargav Kumar Avula, and K. Madhava Krishna, Robotics Research Center, IIIT Hyderabad; and Arun Kumar Singh, University of Tartu, Estonia.
Essentially, PVOs are chance constraints defined over the deterministic velocity obstacles. Thus, a robust MPC formulation is, in fact, an instance of chance constrained optimization (CCO). Recently CCO has been used as a general template for developing numerous navigation algorithms for a wide class of robots, ranging from aerial vehicles to autonomous cars. Although CCO provides a rigorous template for decision making under uncertainty, it is, in general, computationally intractable. In fact, under non-Gaussian or non-parametric uncertainty, it is difficult to even compute an analytical expression for the chance constraints, such as PVO.
In the paper, the authors reformulate CCO as a more tractable problem without making an assumption on the parametric form of the underlying uncertainty and/or resorting to any linearization of the constraints. They show their robust MPC or CCO, in general, can be interpreted as a problem of distribution matching.
Using two different methods, the researchers constructed a certain desired distribution and ensure that the distribution of the PVO matches the desired distribution by choosing low-cost control inputs.
They first proposed a baseline method that approximates the distribution of the chance constraints with a Gaussian mixture model (GMM) and then proceed to perform distribution matching using Kullback Leibler divergence (KLD). Their second method was built on the possibility of embedding distributions in the Reproducing Kernel Hilbert Space (RKHS). The researchers then evaluated both the GMM-KLD and the RKHS based approaches for quality of maneuvers and computational time.
While both approaches can produce safe trajectories, GMM-KLD is highly conservative and leads to poor tracking and control costs. The RKHS-based approach resulted in fewer tracking errors and lower control costs than GMM-KLD. RKHS also provided a massive reduction in computational time.
This is the first work that brings the benefits of RKHS embedding to the domain of robust MPC/CCO; potentially leading to enormous benefits for computationally efficient motion planning and control under uncertainty.
Related Articles:
Helping robots navigate to a target, around obstacles and without a map Which way should I go? 'MorphEyes' stereo camera system improves quadrotor UAV navigation The Falcon and the Flock Alumnus Udit Halder’s work published as cover article in Proceedings of The Royal Society A Levi Burner named a Future Faculty Fellow New algorithms for multi-robot systems in low communication situations Autonomous drones based on bees use AI to work together 'OysterNet' + underwater robots will aid in accurate oyster count Alum Naomi Leonard is 2023 IEEE Control Systems Award recipient
March 12, 2020
|