 |
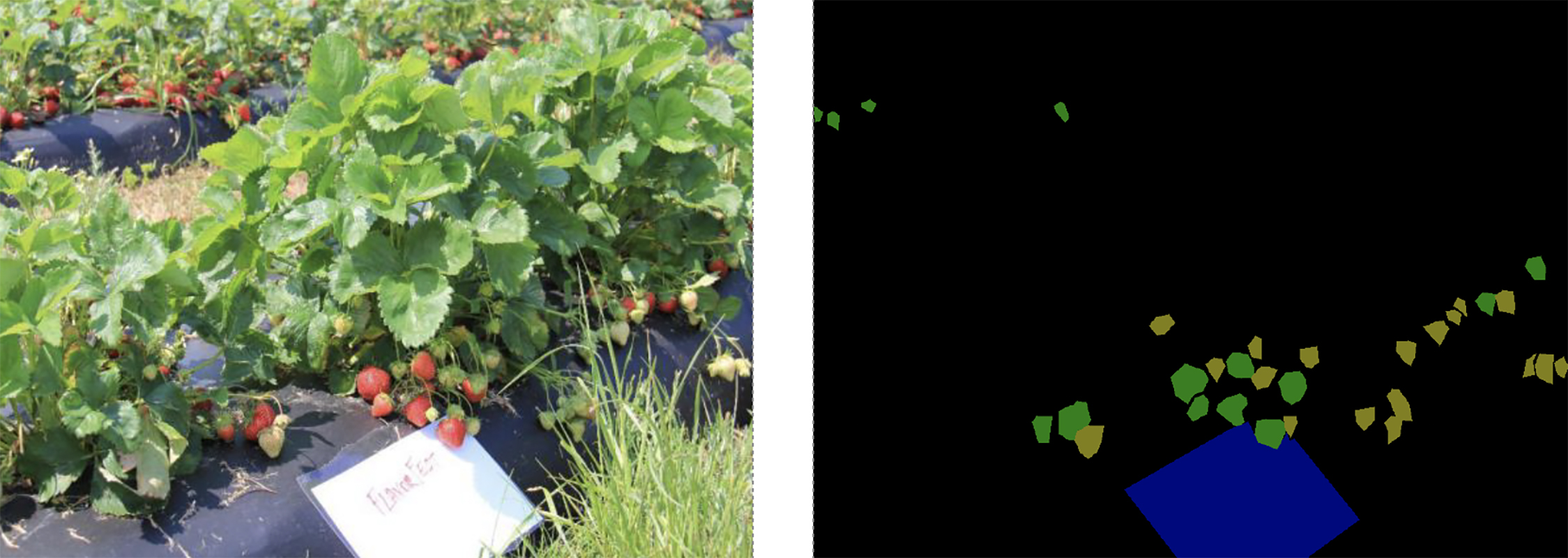
|
 |
Fig. 2 from the paper. Left: Photo of plants in a strawberry field. Right: The corresponding labeled image showing ripe (green) and unripe (yellow) strawberries. |
|
If you’ve ever visited a “pick your own strawberries” farm, you know it can be difficult to find the ripe—but not overripe—fruit. Unfavorable weather conditions like high humidity and excessive rainfall can quickly lead to the strawberries developing rot and disease.
These days, strawberries are on the list of crops that agricultural robots can monitor for better harvesting with less waste. New research by ISR-affiliated Professor Nikhil Chopra (ME), his former student and current postdoctoral researcher Tianchen Liu (ME Ph.D. 2020), and Jayesh Samtani of the Virginia Tech Hampton Roads Agricultural Research and Extension Center uses a combination of robots, computer vision and machine learning to create maps showing strawberries in different stages of ripenes.
Information System for Detecting Strawberry Fruit Locations and Ripeness Conditions in a Farm was presented at the 2022 Biology and Life Sciences Forum.
The researchers developed a farm information system that provides timely information on the ripeness of fruit. The system processes videos and sequences of still images to create ripeness maps of the fields, using state-of-the-art, vision-based simultaneous localization and mapping techniques, commonly known as “SLAM.”
The system generates a map and tracks motion trajectory using image features. First, the images pass through a semantic segmentation process using a learning-based approach to identify the conditions. Then a set of labeled images trains an encoder-decoder neural network model which can determine fruit ripeness based on images. Most ripe and unripe strawberries can be identified correctly, even when parts of the ripe strawberries are covered by leaves. Finally, fruit in different conditions are estimated, helping growers decide when and where to harvest. The system can recommend specific locations within a farm where fruit needs to be picked, as well as places where fruit has rotted or developed disease and needs to be removed.
The system easily can be expanded and calibrated to classify more fruit conditions or to monitor other types of crops. While in this study the images and video clips were collected manually, in the future the system could be used in conjunction with cameras installed on small mobile robots for autonomous data gathering.
Related Articles:
Aloimonos, Sandini contribute chapter to MIT Press book, Cognitive Robotics 'OysterNet' + underwater robots will aid in accurate oyster count Which way should I go? Chahat Deep Singh named a Future Faculty Fellow EVPropNet finds drones by detecting their propellers Bee drones featured on new Voice of America video Perception and Robotics Group creates hive of ideas for drones Zampogiannis, Ganguly, Aloimonos and Fermüller author "Vision During Action," chapter in new Springer book Microrobots soon could be seeing better, say UMD faculty in Science Robotics Deep learning helps aerial robots gauge where they are
August 3, 2022
|