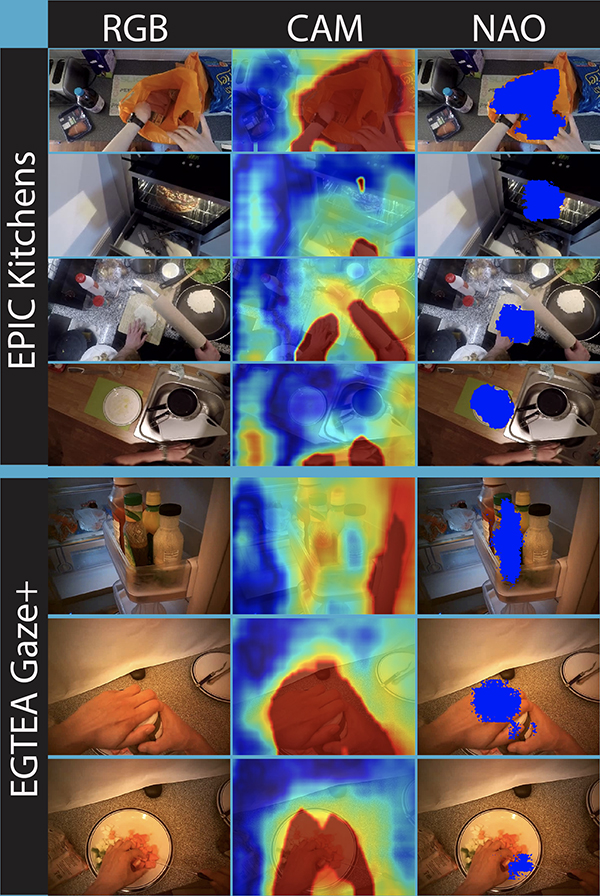
|
 |
Fig. 5 from the paper. The Anticipation Module outputs Contact Anticipation Maps (second column) and Next Active Object segmentations (third column). The Contact Anticipation Maps contain continuous values of estimated time-to-contact between hands and the rest of the scene (visualizations varying between red for short anticipated time-to-contact, and blue for long anticipated time-to-contact). The predicted Next Active Object segmentations contain the object of anticipated near-future contact, shown in blue in the third column. Predictions are shown over the EPIC Kitchens and the EGTEA Gaze+ datasets. |
|
Computer vision uses machine learning techniques to give computers a high level of understanding gleaned from datasets of digital images and videos through automatic extraction and analysis of their useful information. One of the goals of computer vision is to eventually train computers to perform tasks currently done by people. These include automatic inspection during manufacturing, tasks of identification, controlling processes, detecting events, interacting with humans, and navigation—for example the quest to automate vehicles.
Two ISR-affiliated faculty and their students recently used “Ego-OMG” technology they developed to come in second and first in an international computer vision challenge. A paper describing their research, “Forecasting Action through Contact Representations from First Person Video,” was recently published in IEEE Transactions on Pattern Analysis and Machine Intelligence.
The paper was written by Professor Yiannis Aloimonos (CS/UMIACS), Associate Research Scientist Cornelia Fermüller (UMIACS), and their Ph.D. students Eadom Dessalene (CS), Chinmaya Devaraj (ECE), and Michael Maynord (CS). Dessalene is the first author on the paper.
Human visual understanding of action is reliant on anticipation of contact as is demonstrated by pioneering work in cognitive science. Taking inspiration from this, the authors introduce representations and models centered on contact, which they then use in action prediction and anticipation.
They annotated a subset of the EPIC Kitchens dataset to include time-to-contact between hands and objects, as well as segmentations of hands and objects. Using these annotations, the authors trained an Anticipation Module, which produces contact anticipation maps and next active object segmentations. These are novel low-level representations that provide temporal and spatial characteristics of anticipated near future action.
On top of the Anticipation Module the researchers applied Egocentric Object Manipulation Graphs (Ego-OMG), a framework for action anticipation and prediction. Ego-OMG models longer-term temporal semantic relations through the use of a graph modeling transitions between contact delineated action states.
Using the Anticipation Module within Ego-OMG enabled the researchers to achieve first and second place on the “unseen” and “seen” test sets, respectively, in the 2020 EPIC Kitchens Action Anticipation Challenge, as well as achieve state-of-the-art results on the tasks of action anticipation and action prediction.
About EPIC Kitchens
The paper details results obtained using the original 2018 version of EPIC Kitchens, the largest egocentric (first-person) video benchmark dataset, offering a unique viewpoint on people's interaction with objects, their attention, and intentions. EPIC Kitchens features 32 kitchens and 55 hours of recordings that are multi-faceted, audio-visual, non-scripted recordings in native environments.
EPIC Kitchens was created by computer vision researchers from the University of Bristol, England; the University of Toronto, Canada; and the University of Catania, Italy. It is sponsored by Nokia Technologies and the Jean Golding Institute of the University of Bristol, England.
The dataset pushes machine learning towards approaches that target ever more complex fine-grained video understanding. It provides an open-source set of data that helps AI research teams focus deep-learning algorithms and build better computer models for advancing artificial intelligence for the kitchen.
Three challenges were related to the original dataset: action recognition, action anticipation, and object detection in video. These challenges form the base for a higher-level understanding of the participants’ actions and intentions. Researchers may use various components of the videos (audio, for example) to make their systems better at recognizing and predicting actions based on the information from the video. The University of Maryland team entered the Action Anticipation challenge under the team name Ego-OMG. They came in second in the “seen kitchens.” and first in the “unseen kitchens” portions of the challenge.
In July 2020, an updated version of the dataset, EPIC Kitchens-100, was released, featuring 45 kitchens and 100 hours of recording. The new dataset has five associated challenges; the original three (action recognition, action anticipation, and object detection in video), plus domain adaptation for action recognition and multi-instance retrieval.
Related Articles:
CSRankings places Maryland robotics at #10 in the U.S. Autonomous drones based on bees use AI to work together 'OysterNet' + underwater robots will aid in accurate oyster count EVPropNet finds drones by detecting their propellers Zampogiannis, Ganguly, Aloimonos and Fermüller author "Vision During Action," chapter in new Springer book Microrobots soon could be seeing better, say UMD faculty in Science Robotics UMD’s SeaDroneSim can generate simulated images and videos to help UAV systems recognize ‘objects of interest’ in the water Seven UMD Grand Challenges projects include ISR and MRC faculty Levi Burner named a Future Faculty Fellow ArtIAMAS receives third-year funding of up to $15.1M
June 11, 2021
|